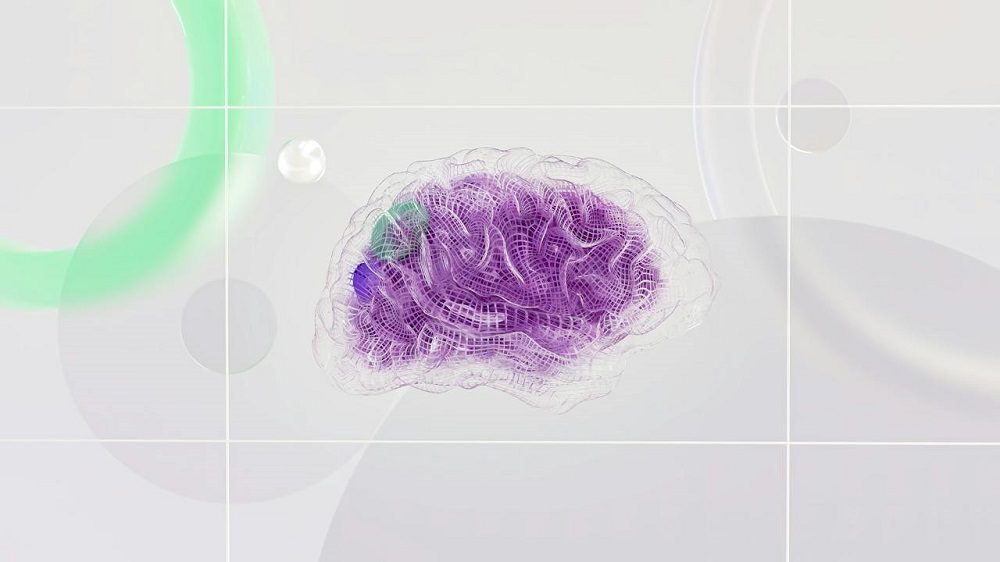
Brain Computer Interfaces: Decoding Words From Brain Signals
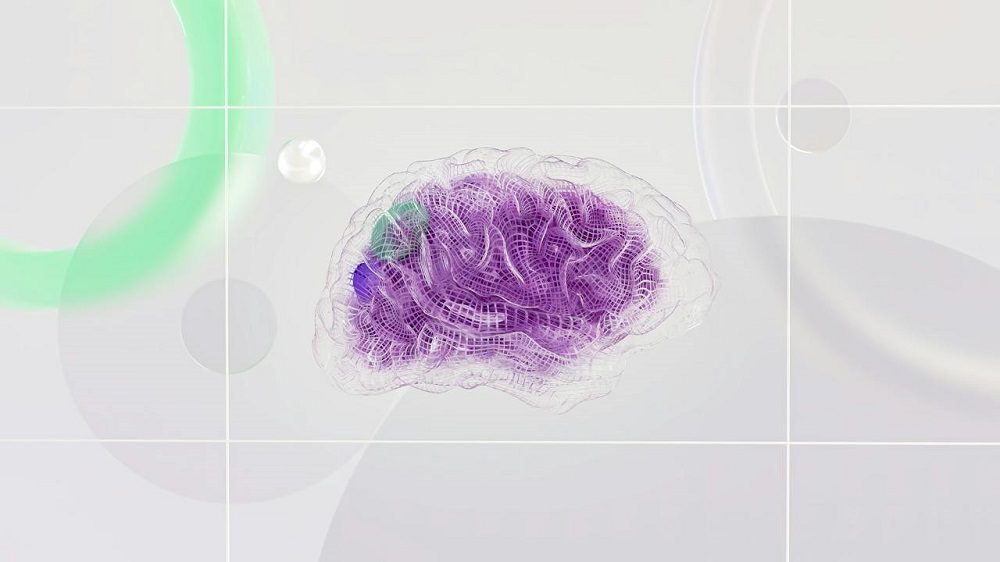
In a groundbreaking development, scientists at Stanford University and the University of California, San Francisco, have unveiled two remarkable brain-computer interfaces (BCI) that decode words from the unspoken but intended speech of individuals who have lost their ability to speak.
These two systems, presented in papers published in Nature, represent significant advancements in speech-decoding BCIs, offering newfound hope and possibilities for individuals who have been speechless due to various medical conditions. This article delves into the details of these cutting-edge technologies and their potential impact on communication.
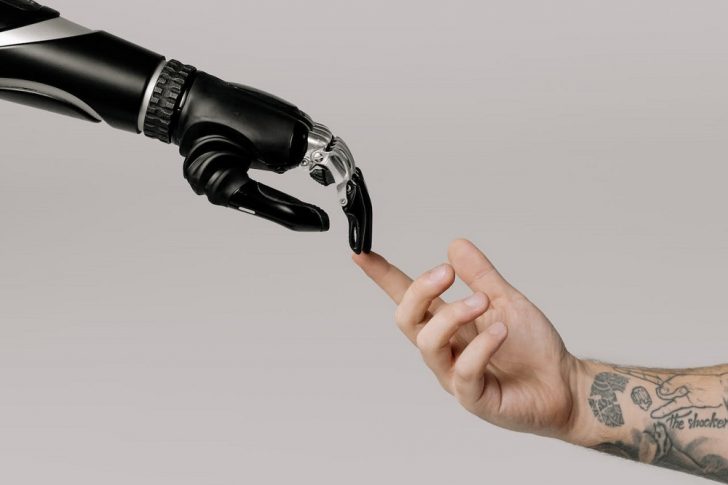
cottonbro studio/ Pexels | The potential for these BCIs to empower individuals like T12 and the stroke survivor is truly remarkable
Unlocking Speech With Brain Signals
Both BCIs have emerged from extensive research conducted with patients facing speech challenges. The first BCI, developed by a team led by Jaimie Henderson at Stanford University, was tested on an ALS patient called “T12” to protect her privacy.
The Stanford team implanted electrode arrays into a specific region of T12’s cortex associated with speech articulation and vocalization. These electrode recordings were then used to train a deep-learning model to associate patterns of neural activity to vocalize individual words.
The two-stage approach first mapped brain recordings to sequences of distinct phonemes, the individual sound units within words, and then combined these sounds into complete words. The result was a digital prosthesis for human speech that translated the intention to vocalize into a series of sounds and, subsequently, known words. This system achieved an average word decoding rate of 62 words per minute, surpassing the previous record of 18 words per minute.
A Potential for Faster Communication Francis Willett, a researcher at Stanford and the first author of the study, believes that T12 could communicate even faster with the device. According to Willett, the device’s algorithm is not the rate-limiting factor, leaving the question of how much faster T12 could communicate with further training.
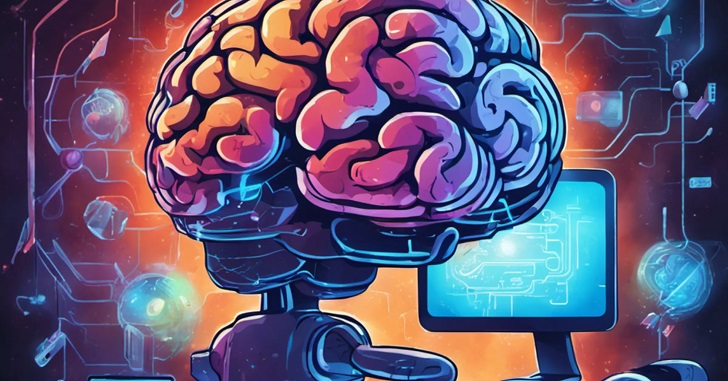
Google DeepMind/ Pexels | The stability of speech decoding through ECoG signals from the brain’s surface is a game-changer
ECoG Array for Stable Speech Decoding
The second paper, authored by researchers led by Edward Chang at UC San Francisco, presents a different approach to decoding speech from brain activity. Their participant, who lost her ability to speak after a brain-stem stroke 18 years ago, used a BCI that converted her neural activity recordings into both text and audio reconstructions of her intended speech.
Unlike the Stanford BCI, this system used electrocorticogram (ECoG) electrodes placed on the brain’s surface, targeting brain areas responsible for the movements of the vocal tract, including the lips, tongue, and jaw.
The UC San Francisco system achieved an impressive output rate of 78 words per minute, outperforming the Stanford team’s device by a significant margin. Moreover, UC San Francisco’s BCI can reconstruct speech in text and audio, enhancing the user’s communication ability.
Stability in Speech Decoding
One advantage of using ECoG signals from the brain’s surface, as highlighted by Sean Metzger, a doctoral student and the first author of the UC San Francisco paper, is its stability in speech decoding.
Unlike signals from individual neurons collected through implanted electrode arrays, ECoG signals do not require daily model retraining. This stability is a significant advantage, ensuring consistent and reliable speech decoding over extended periods.
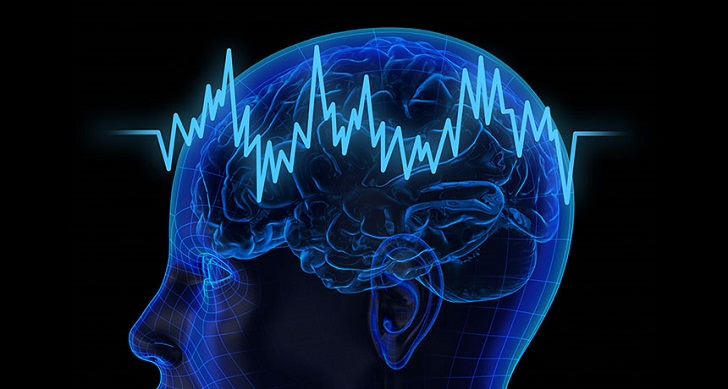
MART PRODUCTION/ Pexels | The introduction of facial avatars to enhance communication adds a deeply human dimension to these BCIs
Humanizing Communication With Facial Avatars
In an intriguing addition to their system, the UC San Francisco team collaborated with the animation firm Speech Graphics to create a facial avatar system. This avatar, representing a human face, mirrors the user’s speech, moving in response to the user’s intended articulations and facial movements.
The machine-learning model controlling the avatar recognizes specific sounds and facial movements from patterns in neural activity. The study participant expressed enthusiasm about this avatar system, suggesting it could aid in her dream of becoming a counselor by facilitating communication with clients through the BCI.
The Path Forward
While both BCIs have made significant strides in speech prosthetic capabilities, they still fall short of the average person’s speaking rate, approximately 160 words per minute. Nevertheless, these breakthroughs mark substantial progress in speech decoding BCIs, offering renewed hope for individuals with speech disabilities.
Both research teams are committed to improving performance and accuracy by exploring the use of more electrodes and enhanced hardware, to reduce word decoding errors in future iterations of their remarkable technologies.
More in Treatment
-
`
5 Reasons Why Dad’s Side of the Family Misses Out
Family bonds are intricate and multifaceted, often creating a unique tapestry of connections. However, many people notice a peculiar trend: stronger...
July 12, 2024 -
`
A Quick Guide on How to Get Short-Term Disability Approved for Anxiety and Depression
Living with anxiety or depression poses unique challenges, particularly in the workplace, where stress can exacerbate symptoms. For many, short-term disability...
July 5, 2024 -
`
Why Do People Feel Sleepy After Eating?
Is feeling sleepy after eating a sign of diabetes? Well, not directly. There are many reasons why you feel drowsy after...
June 20, 2024 -
`
What Is High-Functioning Depression? Symptoms and Treatment
High-functioning depression may not be a term you hear every day, but it’s a very real and challenging experience for many....
June 13, 2024 -
`
Kelly Clarkson’s Weight Loss Ozempic Journey – Debunking the Rumors
In a refreshing moment of transparency, Kelly Clarkson, the beloved singer and talk show host, sheds light on her remarkable weight...
June 3, 2024 -
`
What Is the Best Milk for Gut Health and Why?
In recent years, the milk section at the grocery store has expanded far beyond the traditional options. While cow’s milk has...
May 30, 2024 -
`
Do Dental Implants Hurt? Here’s All You Need to Know
When you hear “dental implants,” you might wince at the thought of pain. But do dental implants hurt as much as...
May 24, 2024 -
`
5 Key Differences Between A Psych Ward & A Mental Hospital
Curious about the differences between a psych ward and a mental hospital? You are not alone. With the mental health conversation...
May 16, 2024 -
`
It’s Official! “Selling Sunset’s” Christine Quinn & Husband Christian Dumontet Are Parting Ways
Have you ever found yourself unexpectedly engrossed in the personal lives of celebrities, especially when their stories take dramatic turns? Well,...
May 9, 2024
You must be logged in to post a comment Login